NativQA
NativQA: Multilingual Culturally-Aligned Natural Query for LLMs
Natural Question Answering (QA) datasets play a crucial role in developing and evaluating the capabilities of large language models (LLMs), ensuring their effective usage in real-world applications. Despite the numerous QA datasets that have been developed, there is a notable lack of region-specific datasets generated by native users in their own languages. This gap hinders the effective benchmarking of LLMs for regional and cultural specificities. In this study, we propose a scalable framework, NativQA, to seamlessly construct culturally and regionally aligned QA datasets in native languages, for LLM evaluation and tuning. Moreover, to demonstrate the efficacy of the proposed framework, we designed a multilingual natural QA dataset, MultiNativQA, consisting of ~72K QA pairs in seven languages, ranging from high to extremely low resource, based on queries from native speakers covering 18 topics. We benchmark the MultiNativQA dataset with open- and closed-source LLMs. We made both the framework NativQA and MultiNativQA dataset publicly available for the community.
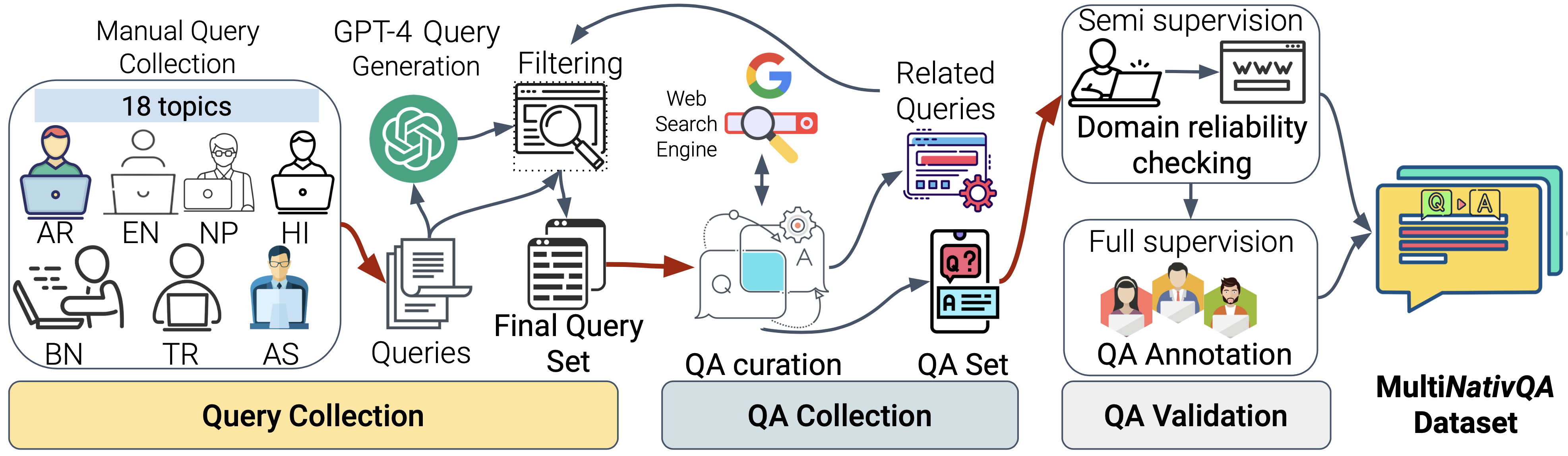
MultiNativQA Dataset
Statistics
TO DO:
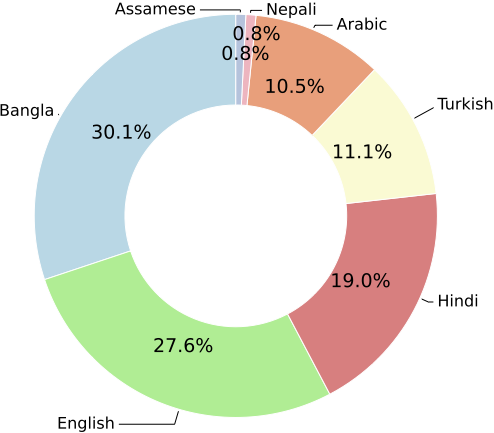
Topics Coverage
Selected topics used as seed to collect manual queries. |
---|
Animal, Business, Cloth, Education, Events, Food & Drinks, General, Geography, Immigration Related, Language, Literature, Names & Persons, Plants, Religion, Sports & Games, Tradition, Travel, Weather |
Language Coverage
news
No news so far...
latest posts
Jul 16, 2024 | Arabic Language Technologies – Medium |
---|